OSMnx is a Python package for easily downloading and analyzing street networks anywhere in the world. Among other analyses, we can use it to explore street network orientation. That is, what are the bearings and spatial orientations of the streets in the network? All of the code for this example is in this GitHub notebook. First we use OSMnx to download the street network of Moraga, California, a small town in the hills just east of Berkeley:
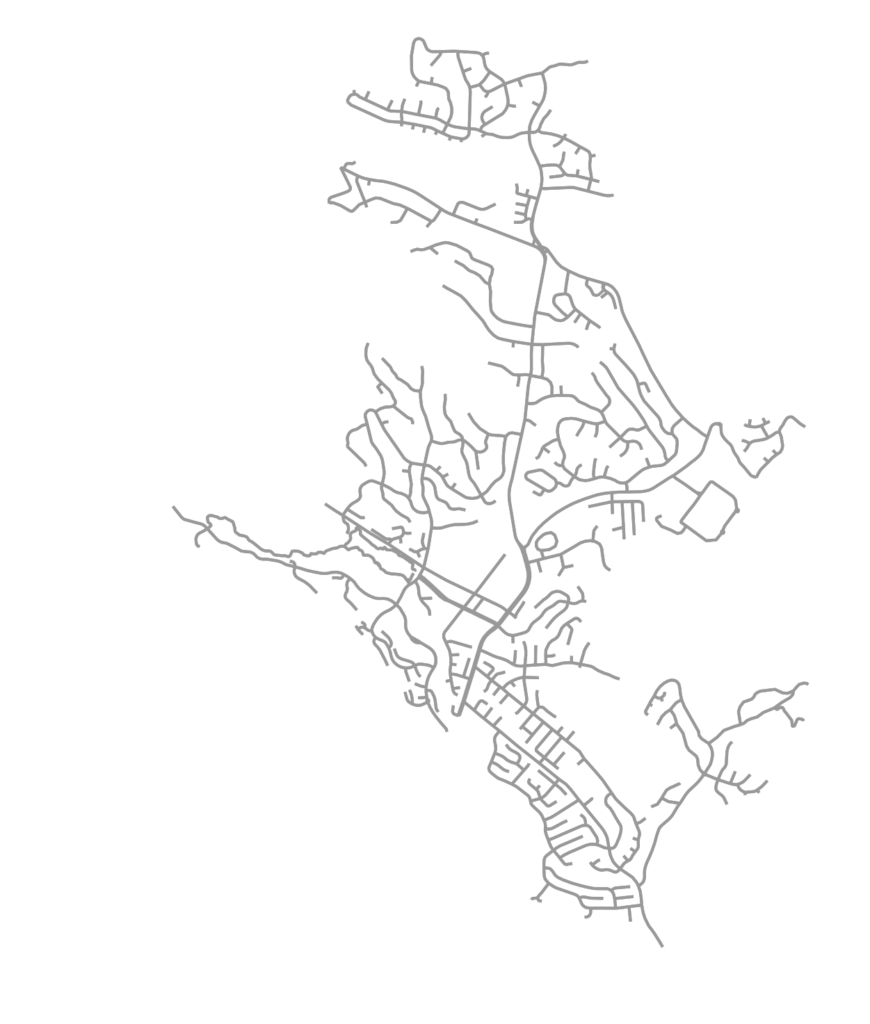
OSMnx automatically calculates all of the streets’ bearings. Specifically it calculates the compass bearing from each directed edge’s origin node u to its destination node v. Now we can visualize these bearings, binned together as a histogram to get a sense of the relative frequency of the streets’ spatial orientations. Or better yet, we can project that histogram as a polar plot to match the compass bearings:
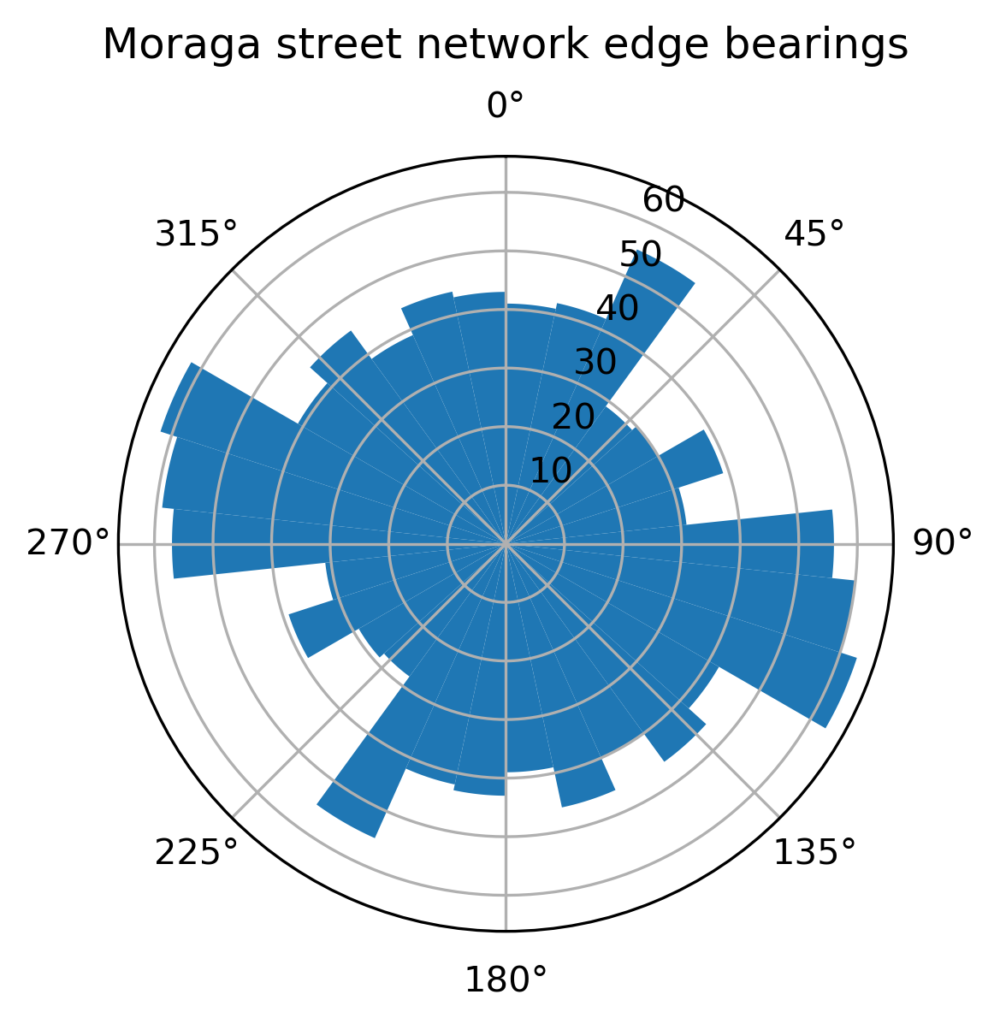